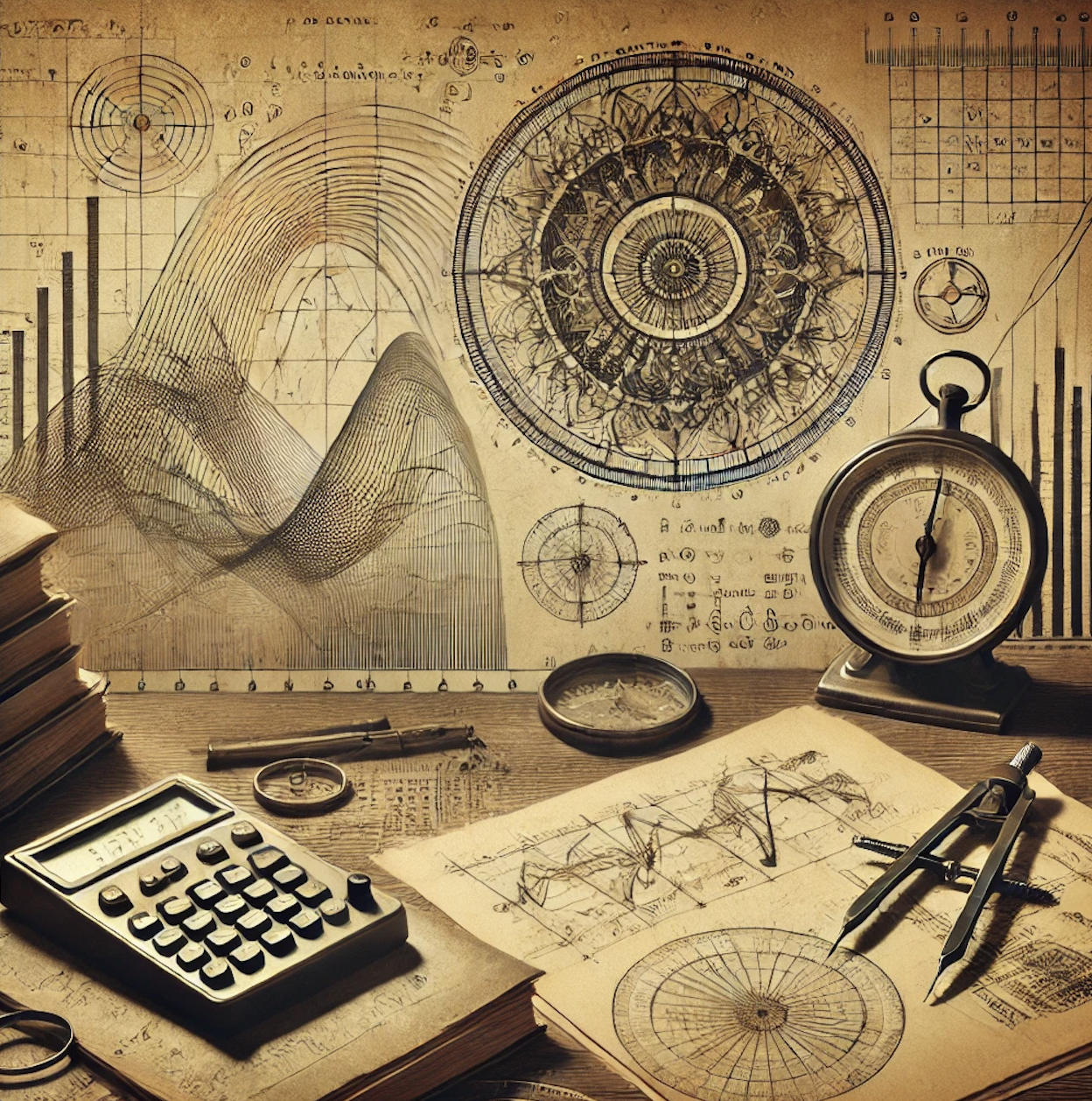
Another reason we need Bayesian models and experiments in marketing mix models
In a brilliant paper currently in pre-print called Your MMM is Broken: Identification of Nonlinear and Time-varying Effects in Marketing Mix Models, the authors describe an interesting challenge (to add to the already quite long list).
The paper highlights why standard MMMs can generate misleading results due to a conflation between diminishing returns and time-varying effects, and how Bayesian models and experiments can overcome these issues.
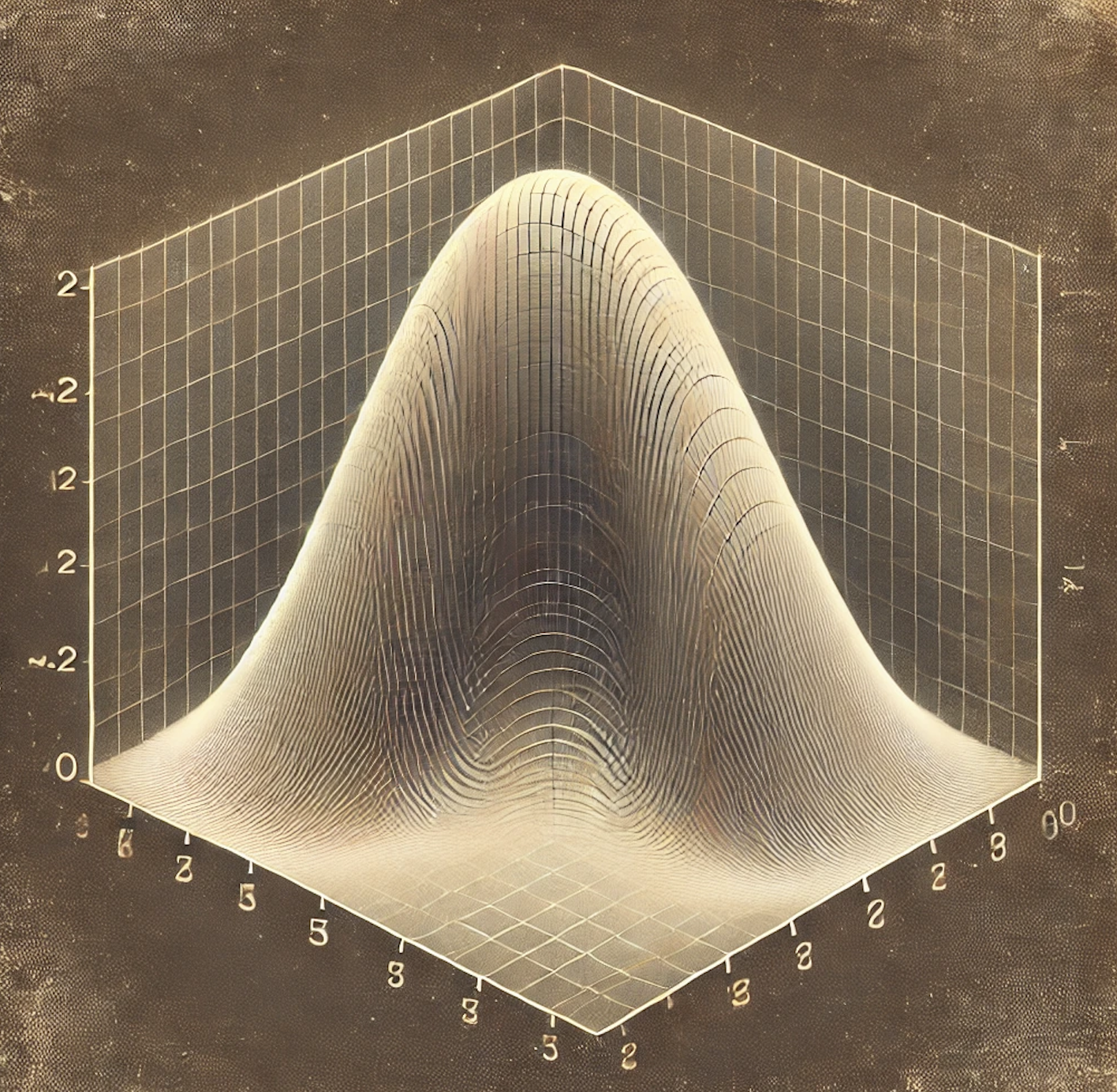
How to measure customer lifetime value
Customer Lifetime Value (CLV) is a fundamental business metric, yet many organisations struggle to measure it accurately. This is often due to simplistic approaches rather than a lack of adequate data. In this post, I outline key principles for measuring and building trust in CLV estimates—an essential step toward informed, customer-centric decision-making.
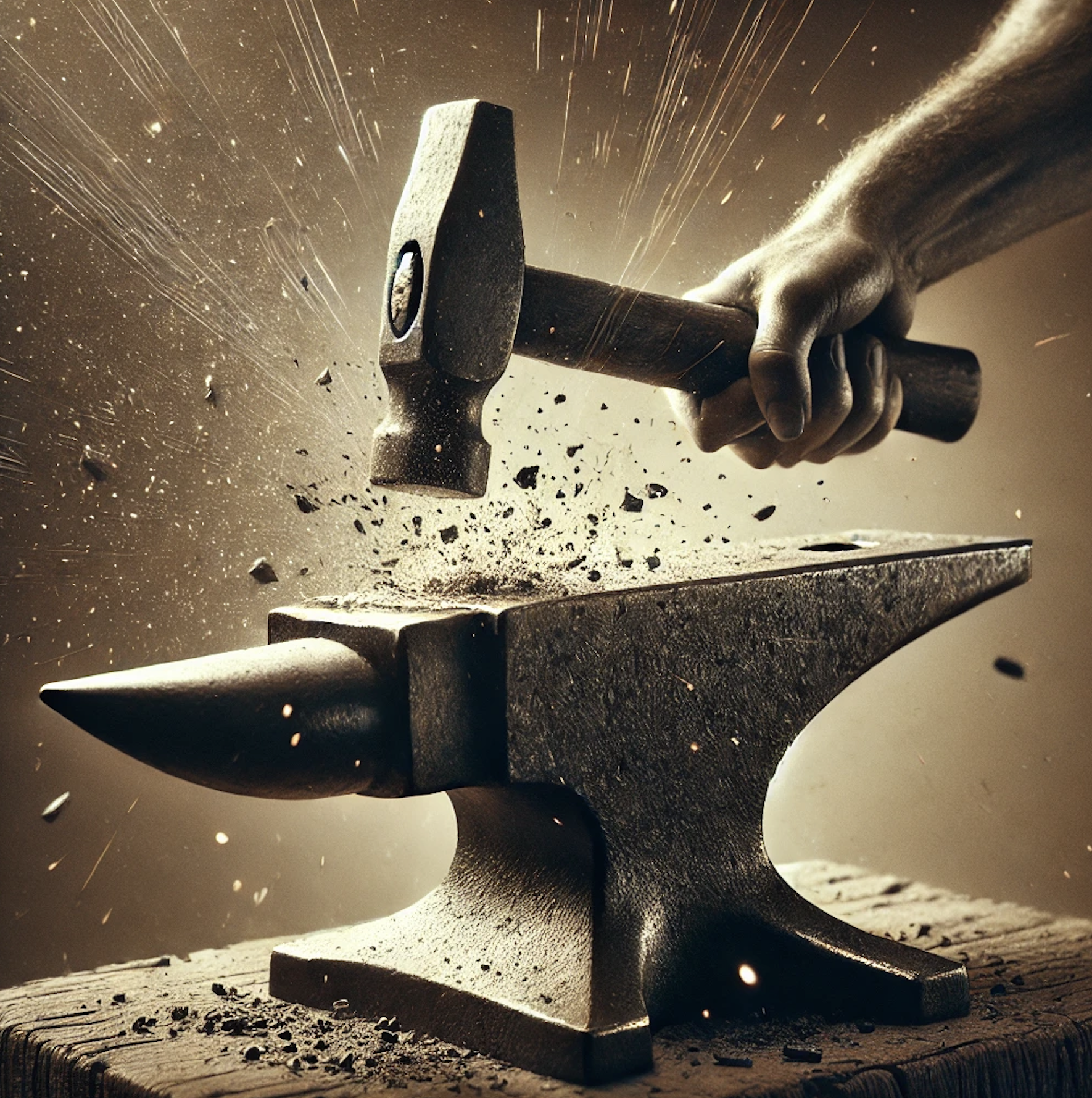
Bayesian MMM myth busting
In the process of writing ‘Making Effectiveness Work’ for the IPA, I came across a lot of misleading commentary on the challenges (and benefits) of Bayesian approaches to MMM. Here are 5 of them…
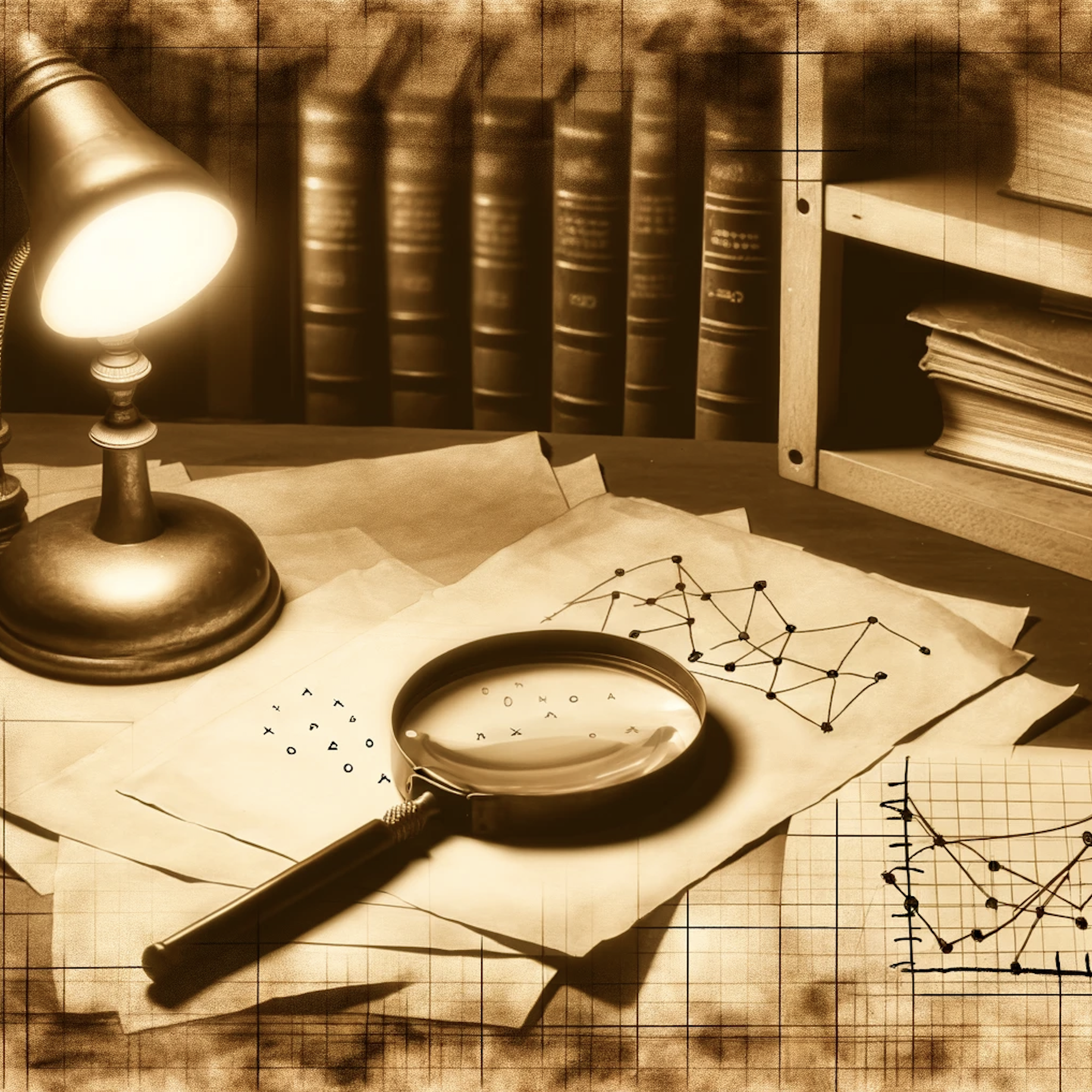
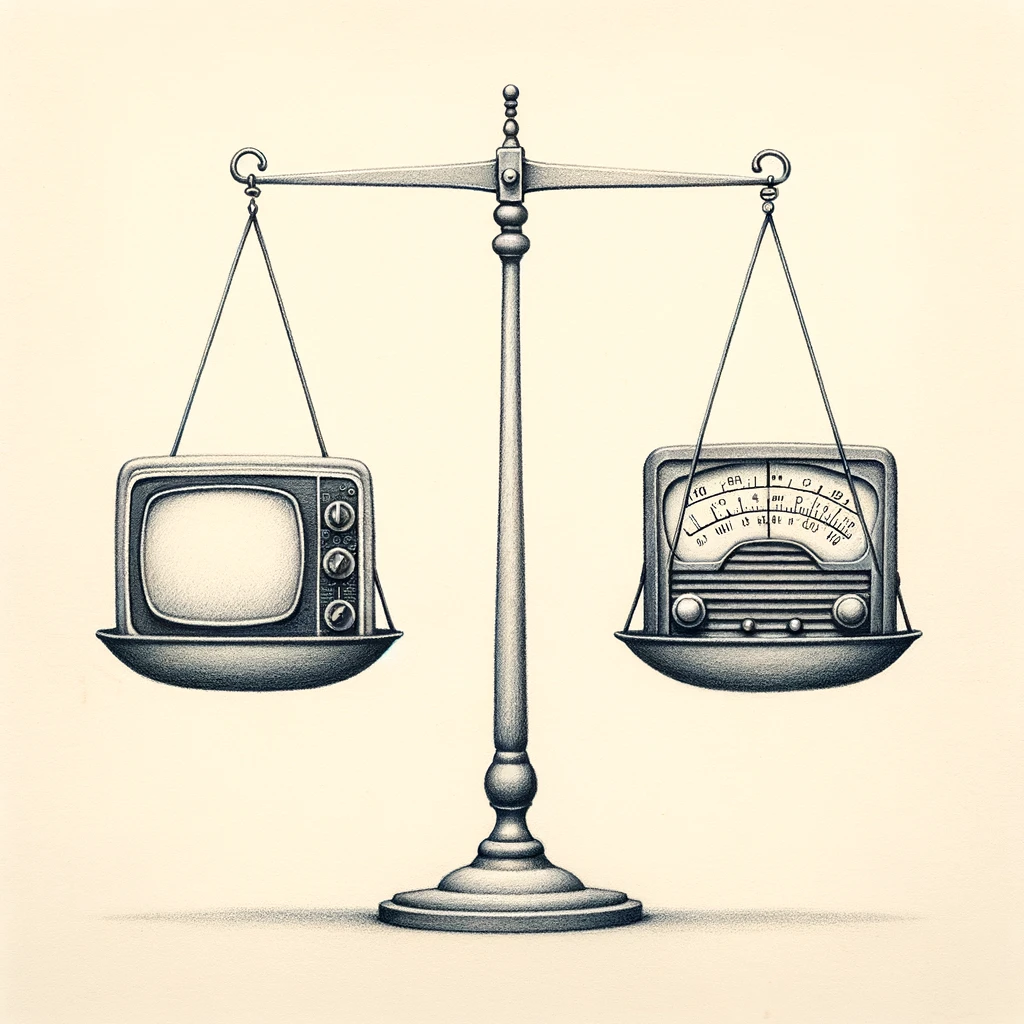
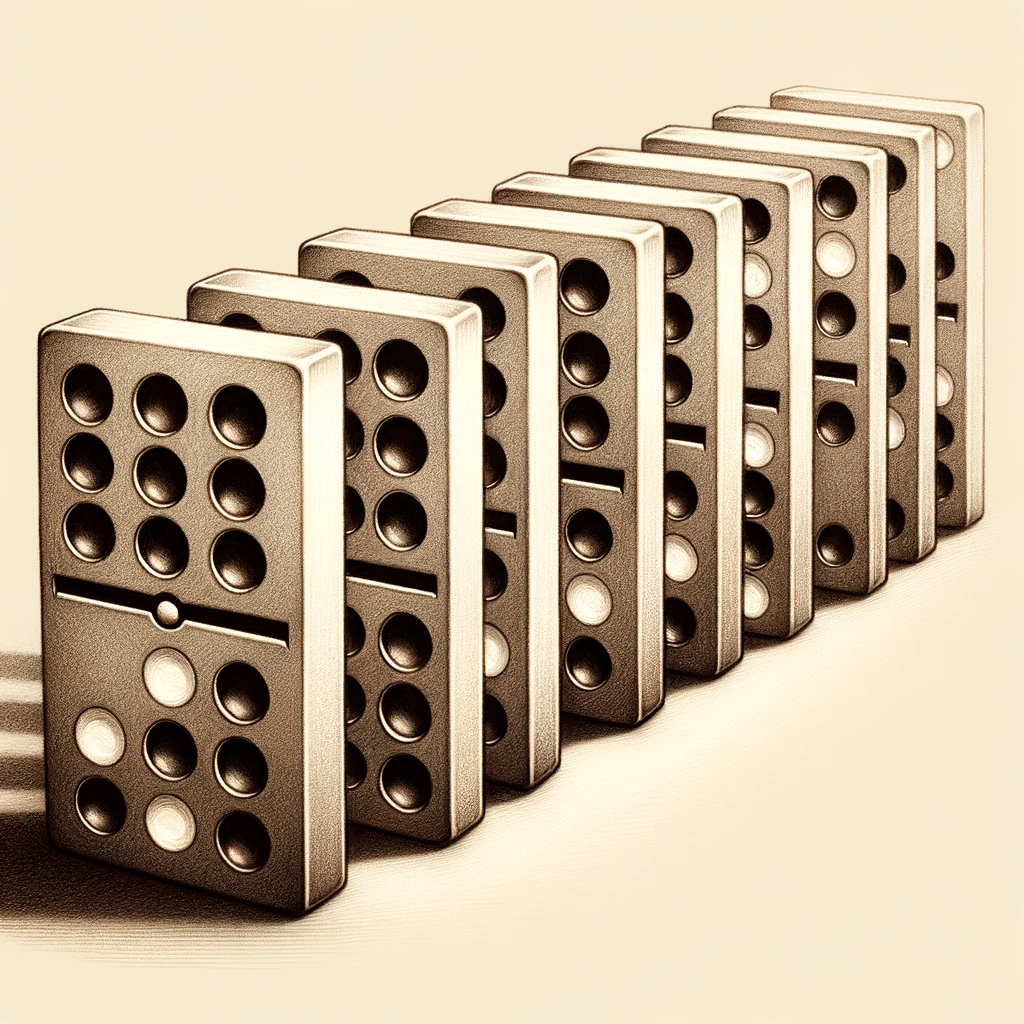
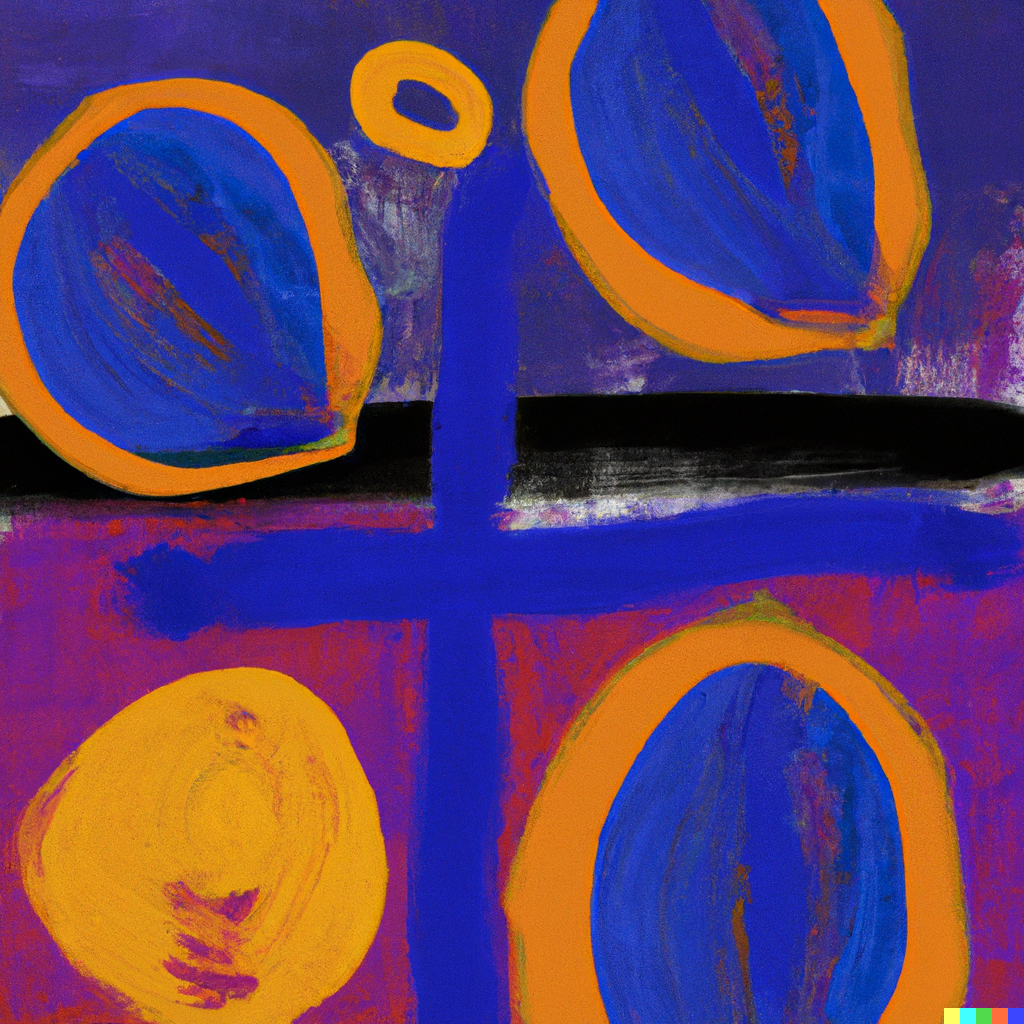
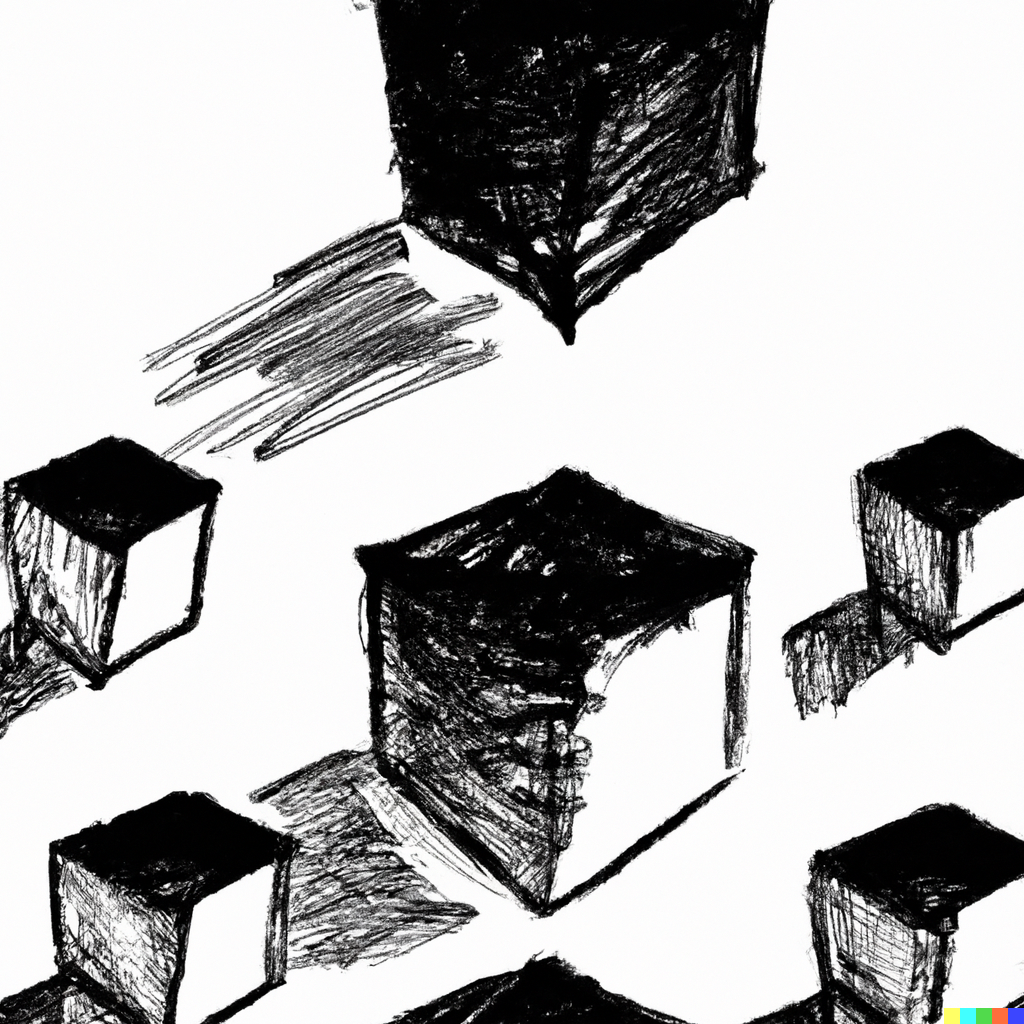
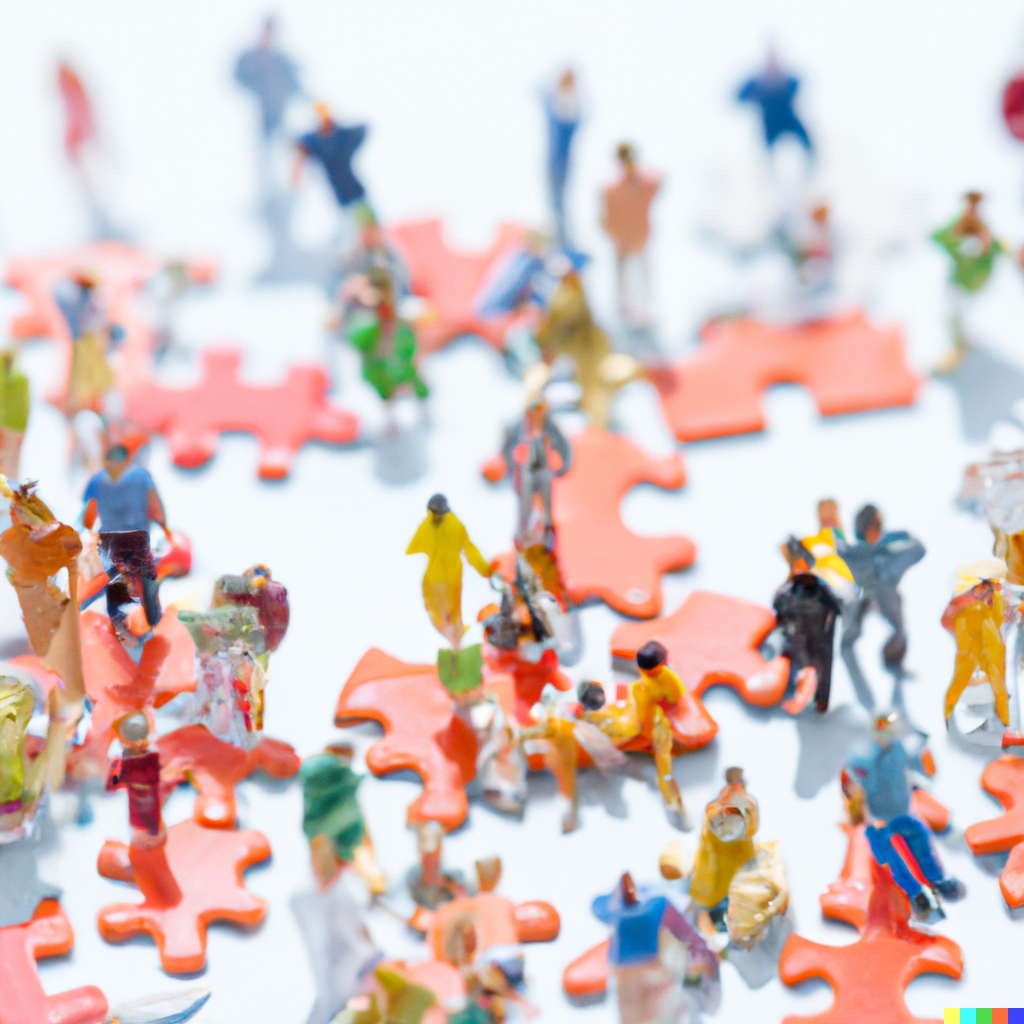
Unlocking the Power of Customer Lifetime Value: 5 Key Benefits for Your Business
Accurately measuring customer lifetime value allows companies to grow profitably by acquiring higher value, committed customers at a lower cost; by ensuring the profitable ones stay customers for as long as possible; and by developing your relationship with them to ensure they engage with your brand as much as possible.
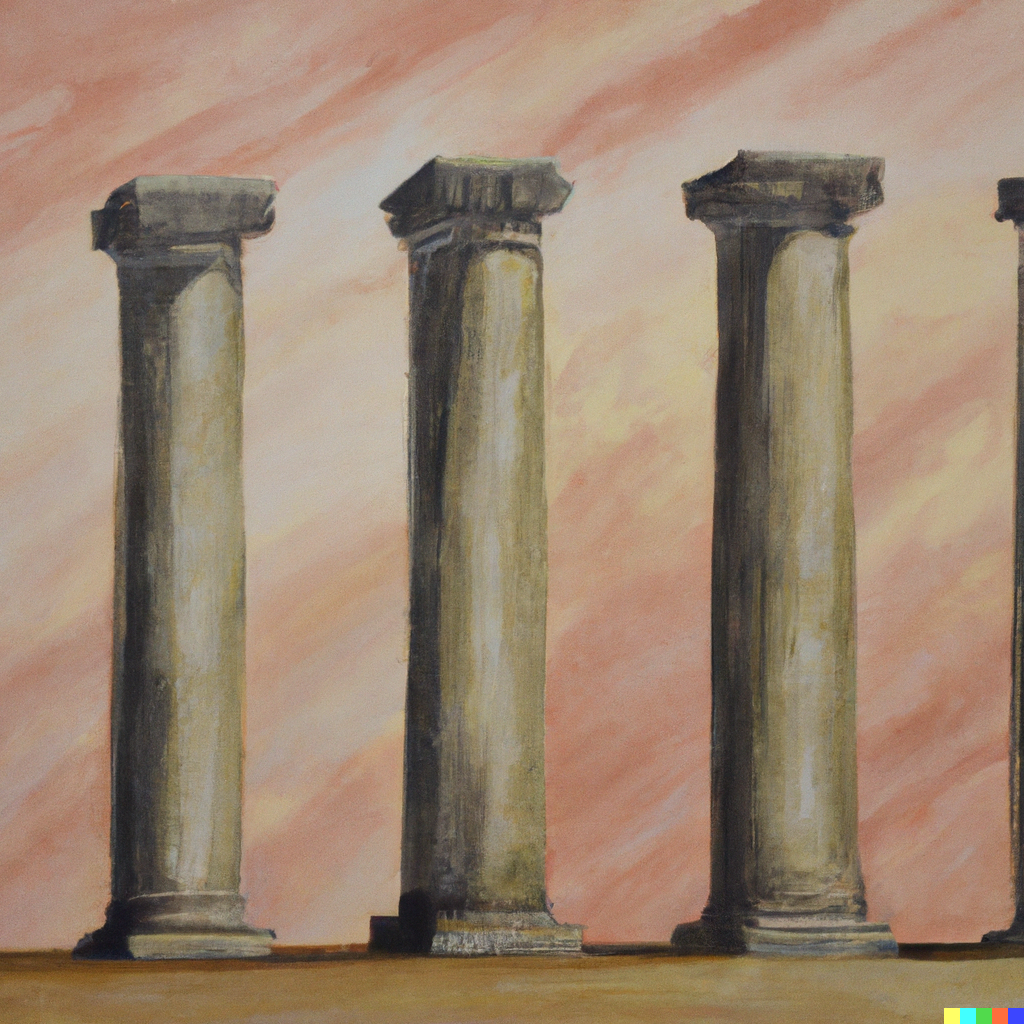
5 pillars of good marketing measurement
The resurgence of interest in MMM presents an opportunity to shake up marketing science, organising measurement around the critical decisions marketers face.
But many providers are burrowing deeper into existing rabbit holes - spending huge amounts of time and money building overly complex, granular models, which produce results that ultimately have a limited impact on decision making.
A better approach is to focus analytics to where it can have the most impact and to organise measurement around business-critical decisions.
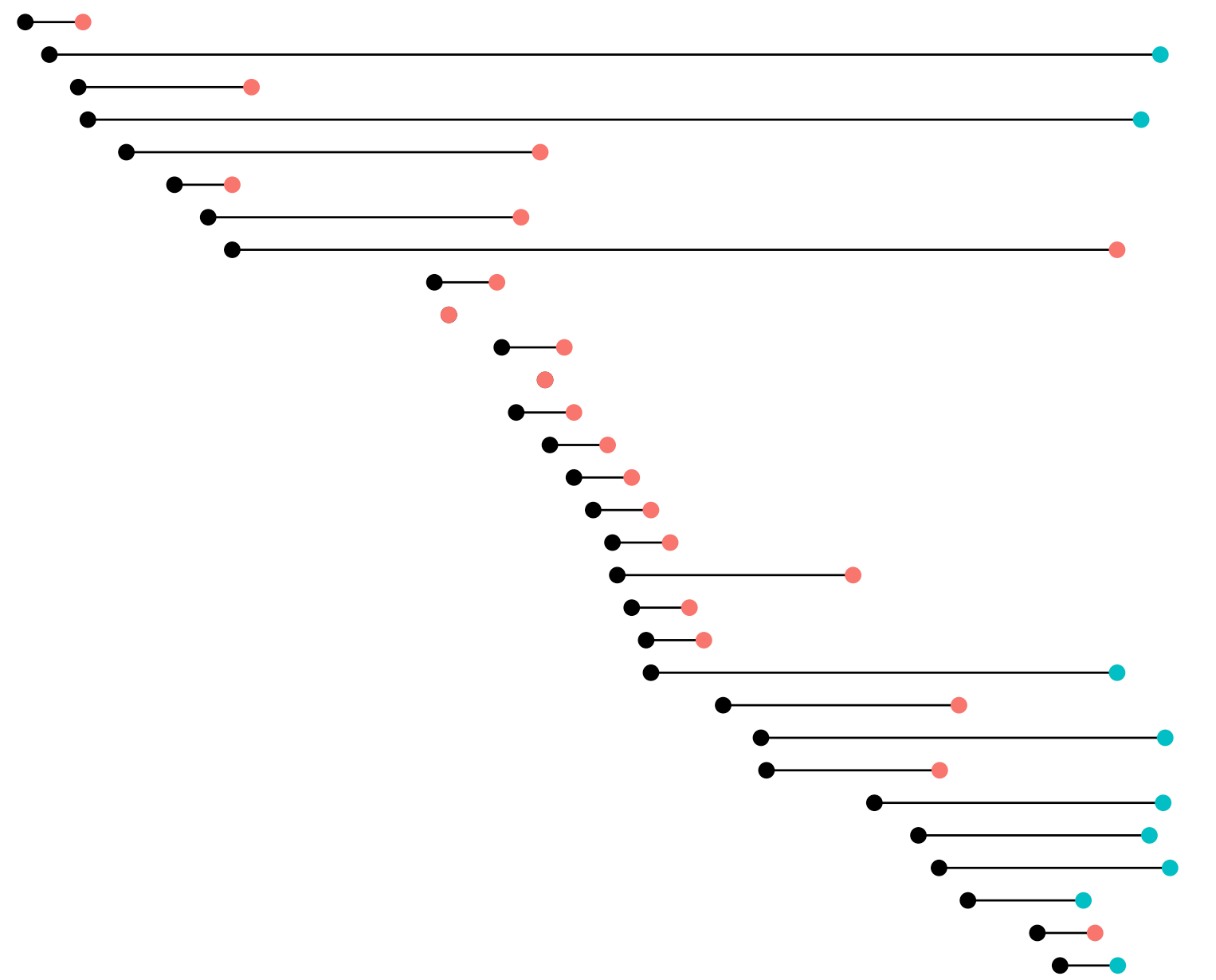
Predicting subscriber lifetime value using survival analysis
How to predict subscriber retention and estimate lifetime value
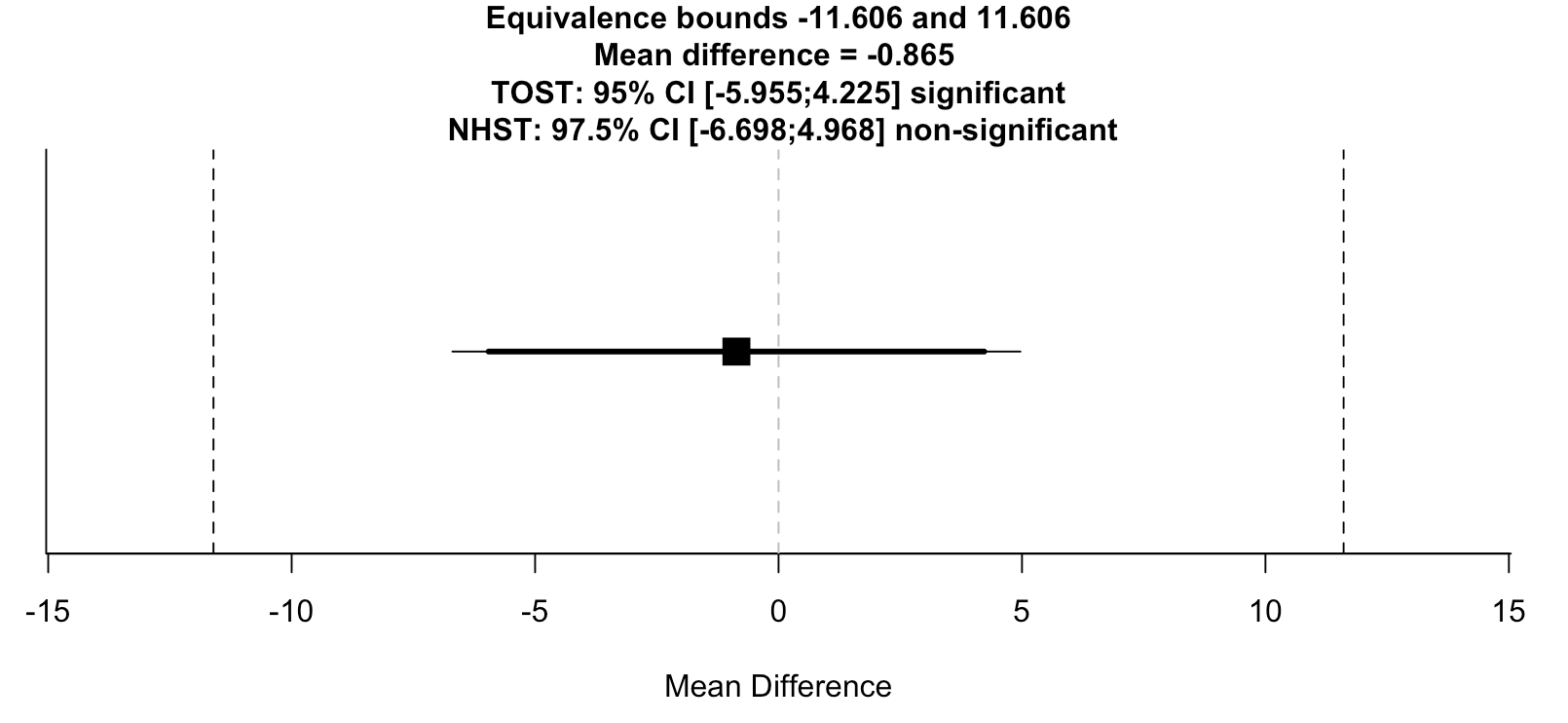
New publication looking at the impact of point-of-care genotyping on routine clinical practice
We collaborated with researchers at the Manchester Centre for Genomic Medicine on a paper looking at the impact of introducing point-of-care genotyping on routine clinical practice.
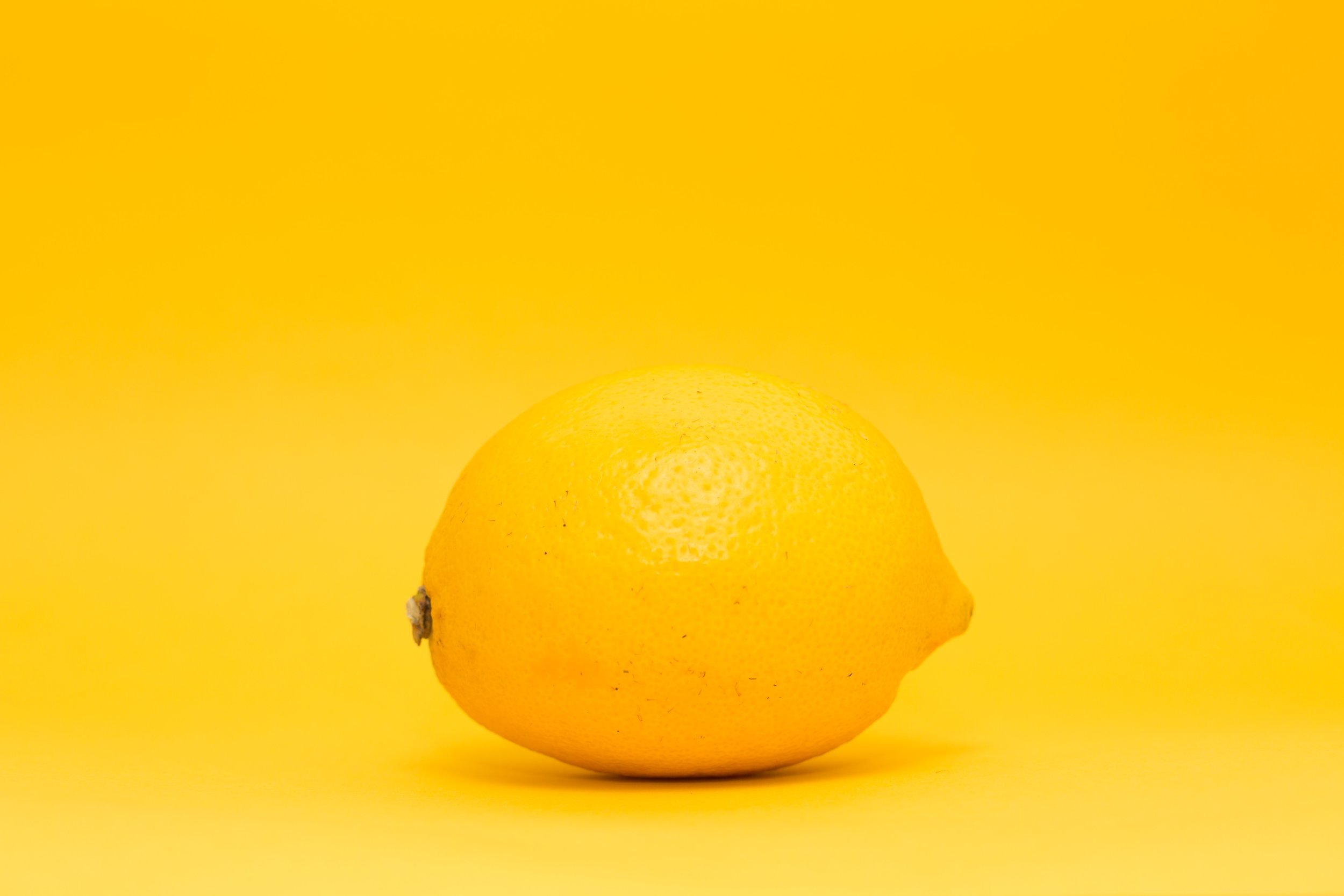
Is data science becoming a market for lemons?
Is data science becoming a market for lemons?
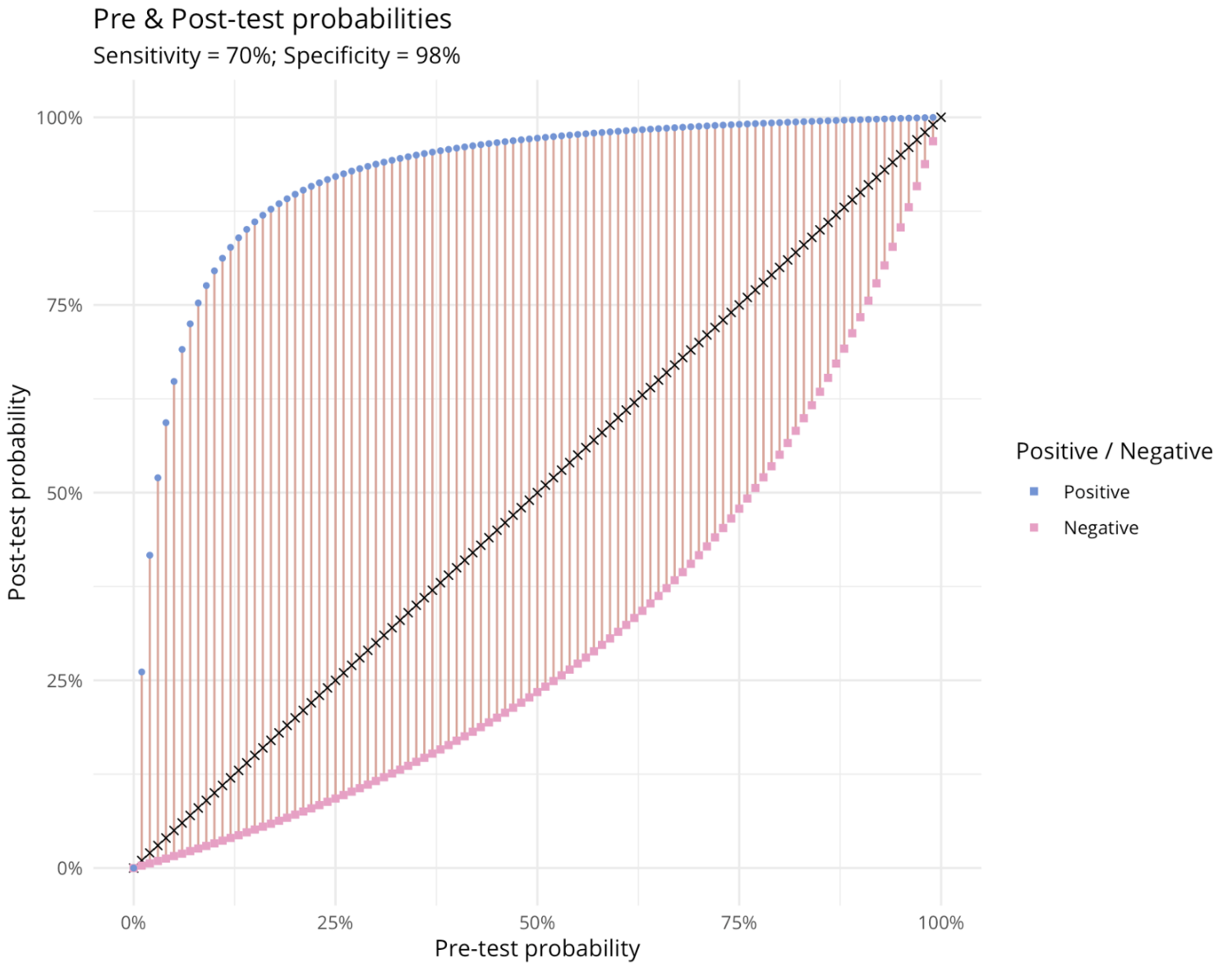